A sneak peek into the book
What makes AI click, what makes it snake oil, and how to tell the difference
In April 2022, many images like this one went viral. What was special about it was that it was generated using a text-to-image AI system called DALL-E 2, using just a one-line description: “A photo of an astronaut riding a horse”.
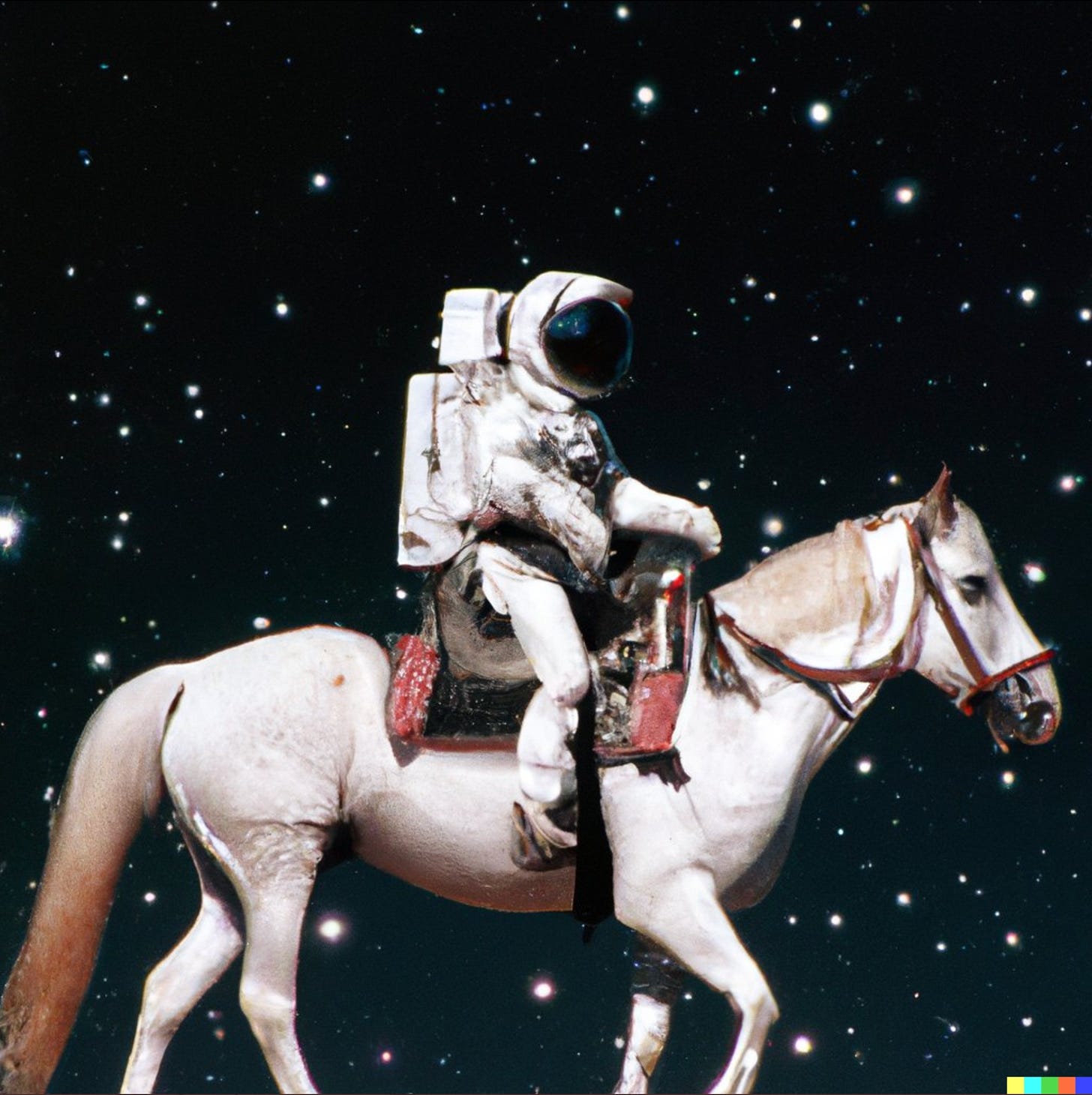
Tools such as DALL-E 2 and Google’s Imagen have made stunning improvements in creating realistic-looking images based on one-line prompts.
In May 2016, ProPublica published a shocking report showing that COMPAS, a tool used to predict whether a defendant would commit a crime if released before trial, was twice as likely to falsely accuse a Black defendant compared to a white one.
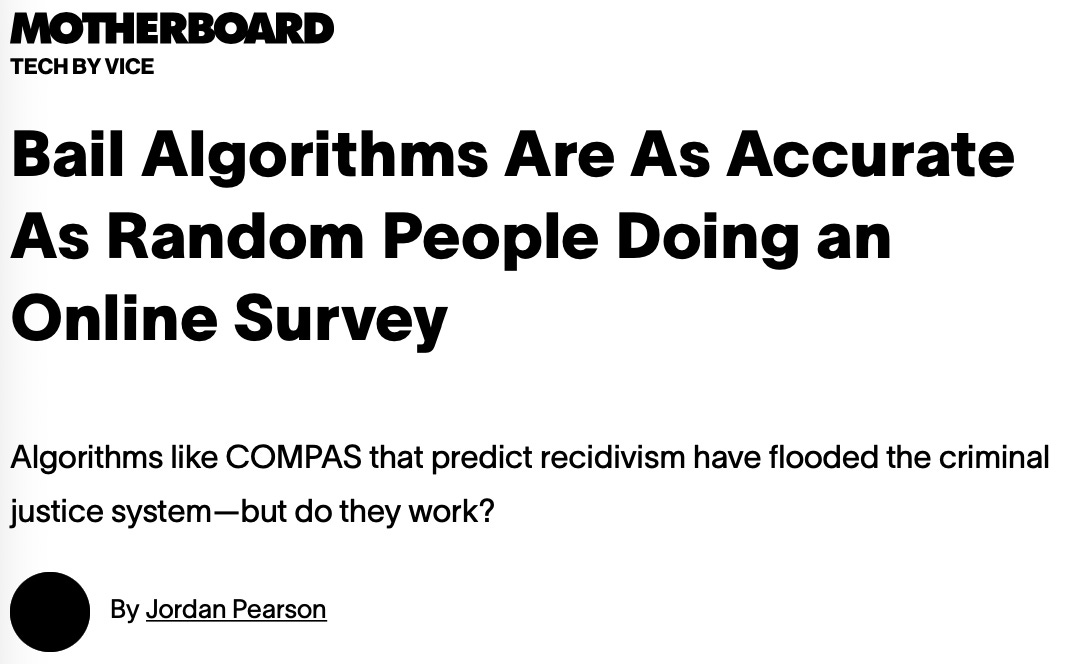
More worryingly, researchers found that COMPAS, which used hundreds of data points about a person to make a decision, was not very effective at predicting the future at all—it was as good as leaving the decisions up to people with no background in criminal justice. In fact, COMPAS was no better than using just two pieces of information to predict if someone would commit a crime: their age and the number of prior offenses!
Both DALL-E 2 and COMPAS work by learning patterns from data, and can be thought of as AI. Why is AI so good at creating images from text, and yet so bad at predicting who will commit a crime?
AI is an umbrella term. Today, it is used to refer to a large number of related but different applications. Some of them have made massive progress, such as DALL-E 2. Others, such as COMPAS, do not work—and perhaps never will. So how can we understand which AI systems can work and which ones cannot?
That’s where our book comes in. We explore what makes AI click, what makes certain problems resistant to AI, and how to tell the difference. AI is everywhere today, so this is essential knowledge if you ever make decisions about AI-powered products or services, whether at work or in your personal life. It is also essential for policymakers, journalists, and many others.
How AI has gotten so good in some areas
Our phones have apps that can instantly recognize which song is playing in the background or transcribe our speech fairly accurately. Machines have trounced the world champion at games such as Go. None of these was possible a decade or two ago. What do these applications have in common?
AI has made massive progress in applications where there is little uncertainty or ambiguity. For instance, when training a face recognition model, the model uses labels that tell it whether any two photos represent the same person or not. So, given enough data and computational resources, it will learn the patterns that distinguish one face from another.
Though there have been some notable failures of face recognition, it continues to get much more accurate (and that’s exactly why we should worry).
Similarly, the game Go has clear rules, and we can generate as much data as we want by just letting the machine play against itself. Again, the lack of ambiguity and the abundance of data allow AI to get better at playing Go.
But the progress that’s been made in face recognition or Go-playing does not transfer to other domains. Limitations of AI are amplified when there are no clear rules, when collecting additional data is hard or impossible, and when reasonable people can disagree about the right answer.
AI is not a magic 8-ball
Given enough data and computational resources, is everything predictable?
In 2017, our colleagues at Princeton University tried to answer that question. They organized a prediction competition: using over 10,000 data points about children, collected based on hours and hours of surveys and in-depth interviews with each family, hundreds of researchers aimed to predict how well each child would do in the future.
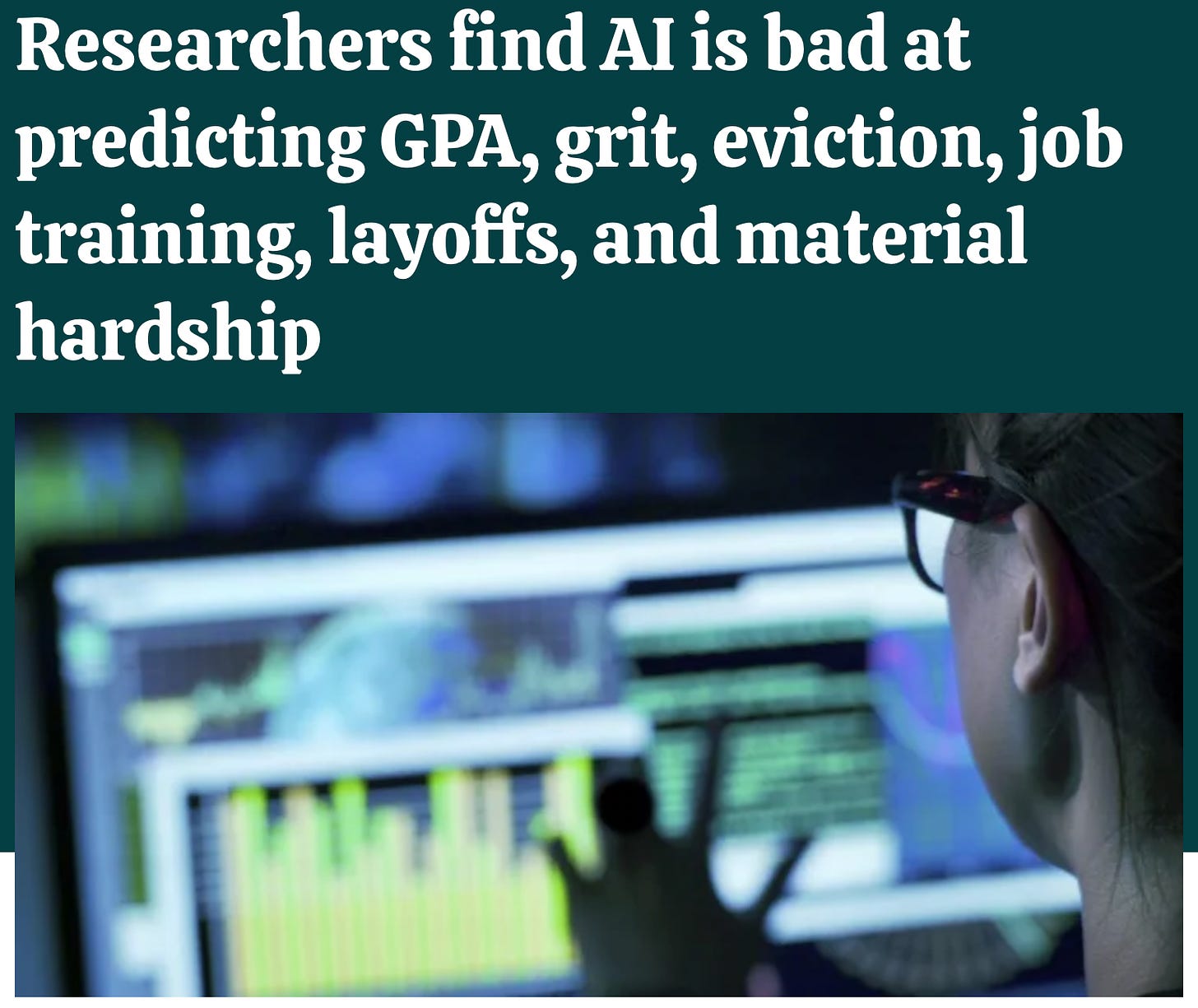
They were massively disappointed to find that the latest AI techniques barely performed better than simple linear regression and just four pieces of information about the children, such as race and mother’s education level. None of the models could predict future outcomes very well, reminiscent of COMPAS.
There are many reasons why predicting the future is hard: peoples’ lives could face sudden shocks, such as getting laid off or winning a lottery, that no model can predict. Small changes in a person’s life, such as one visit to the emergency room, could have compounding effects on their future, for instance, due to large medical bills.
Yet, many companies and developers claim that their products can predict the future. For example, HireVue claims to predict future job performance using questions such as “Is your desk busy or minimal?” to infer applicants’ personalities. That’s basically a horoscope, yet this tool decides the fate of millions of people every month.
HireVue is not alone in claiming to predict individuals’ futures: EAB Navigate claims to predict which students will drop out of college and the Allegheny Family Screening Tool claims to predict which children are at risk of maltreatment. Each of these tools is an attempt to use AI to predict social outcomes of interest.
However, unlike speech transcription or image generation, there is no ground truth here, since the outcomes being predicted are in the future—they haven’t happened yet. On top of that, it is often impossible to collect the kinds of data required to make good predictions.
We think many applications of AI for social prediction are Snake Oil—they don’t work and perhaps will never work.
Can good judgment be automated?
In April 2018, Mark Zuckerberg was grilled by the U.S. Congress about what Facebook was doing about objectionable content such as troll accounts, election interference, fake news, terrorism content, and hate speech. His answer in all these cases was that AI tools would take care of it. The policymakers appeared to mostly accept his answers at face value, and didn’t know enough about tech and AI to call him out.
Content moderation is an example of what we call “automating judgment.” Why do we think AI won’t solve the problem? Since Facebook records the judgment of human moderators on millions of pieces of content, shouldn’t it be able to automate their work by training a model to recognize the patterns in their decisions?
There are two main barriers. The first is that AI remains notoriously bad at nuance and context. Google’s algorithms reported a man to the police and terminated his account — causing him to lose access to more than a decade of contacts, emails, and photos — because he took a picture of his toddler’s infected genitals to send to the doctor. Predictably, the algorithms mistook this for child sexual abuse imagery. There are thousands and thousands of stories like that one.
Even if these problems can someday be overcome, there’s a much deeper one. AI can’t make policy, only implement it. Once human moderators have already labeled thousands of examples on either side of the line between acceptable and unacceptable nudity, or acceptable speech and calls to violence, AI can learn where the line is. But the hard part is drawing the line. New examples come up all the time that test our understanding of where the line is. This requires judgments based on our values. In the hard part of content moderation, AI does not and cannot have a role.
In our chapter, we’ll elaborate on these arguments and show you many more reasons why AI’s potential for automating judgment isn’t what it’s cracked up to be. These reasons apply not just to content moderation, but many other problems that at first seem very different but share some essential features, ranging from preventing imminent self-harm to automated essay grading (yes, that’s a thing, and it’s widespread today).
Why do myths persist?
If AI can only work in a narrow domain of tasks, why do myths about AI persist?
Researchers, companies, and the media can (even unwittingly) collude to create hype about AI, and public understanding of AI is collateral damage.
Hundreds of thousands of papers on AI are published every year, and most claim to make some advances. How many of these can be trusted?
A cornerstone of scientific progress is the ability of independent researchers to verify previous results. Worryingly, research in AI falls short. For example, out of 400 recent papers in top AI conferences, none documented their methods in enough detail such that an independent team could verify that their research was correct.
In addition, many scientific fields such as radiology, psychology, and economics have also begun to adopt AI. However, AI methods are tricky to use correctly, and when errors occur, they are hard to detect. As a result, there are widespread errors due to adopting AI in at least 17 different fields (and likely in many more!). This is in part because a misplaced trust in AI leads to lesser scrutiny of flashy results.
Just as researchers have incentives to make overhyped claims about AI, so do companies. In fact, not having to publish peer-reviewed research, nothing stops companies from making unsubstantiated claims about the effectiveness of their AI products. This works. Research has shown that venture capitalists who fund startups and clients who buy AI tools are swayed by these claims and don’t inspect them too carefully.
Worse, companies exploit the fact that AI is an umbrella term without a precise definition. Whatever it is that they are selling and whether it works or not, claiming that it uses AI helps sell it — even when it’s humans behind the scenes! In other words, companies both capitalize on and contribute to public confusion about AI’s capabilities and limits.
Commercial AI hype is so successful because it is amplified by the media. Newsroom budgets have been decimated, so it’s always tempting for journalists to simply rewrite press releases, add a quote, and hit ‘publish’. Sensationalist headlines and misleading stock photos take the hype to the next level.
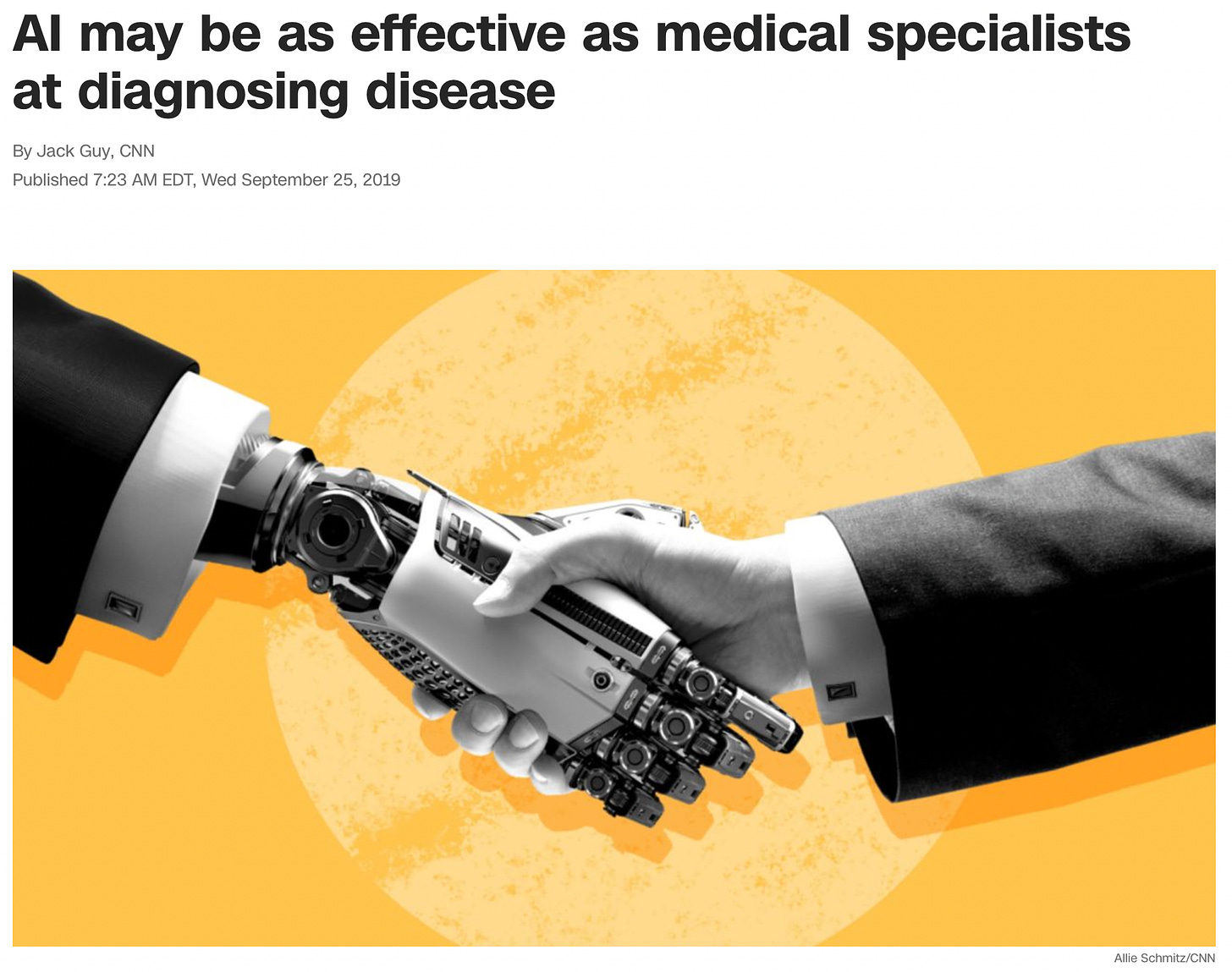
Bombarded with hype from researchers, companies, and journalists, it’s hard for any of us to evaluate claims about AI critically. On top of that, we share cognitive biases that make us especially susceptible to hype. For example, we tend to anthropomorphize AI—treat it as if it is an agent with human-like qualities. That leads to misplaced trust in AI systems.
Cognitive biases can also prevent us from recognizing the limits of our own knowledge. For instance, we are often overconfident in our understanding of how things work—we feel that we understand complex phenomena in more detail than we actually do.
All of these result in a warped understanding of AI progress. The public is ready to believe that there is a high likelihood of AI achieving human-level intelligence in the next 10 years!
Because people are awed by this technology, there’s not nearly enough resistance when flawed, black-box AI is deployed in hugely consequential situations, such as in criminal justice or hiring, with no opportunity for people to challenge decisions made about them.
What can be done about AI snake oil?
Obviously, we think researchers and companies need to do better at avoiding hype. But we’re not holding our breath. The problem is that the incentives favor bold and unverifiable claims, and there are few consequences for misleading the public.
In 2016, AI pioneer Geoffrey Hinton claimed: “If you work as a radiologist, you're like the coyote that's already over the edge of the cliff but hasn't yet looked down, so he doesn’t realize there’s no ground underneath him. People should stop training radiologists now. It's just completely obvious that within five years, deep learning is going to do better than radiologists.” Six years later, deep learning or any other form of AI has not even come close to replacing radiologists. AI for healthcare has been failure after failure. Asked about this in 2022, Hinton claimed he never predicted that AI would replace radiologists.
Journalists and advocates can play a role in shifting these incentives. Consider the issue of AI bias. Over the last five years, a loose community of scholars and activists has had considerable success in changing the narrative that AI is just math, and thus unbiased. People now understand that AI will, by default, reflect society’s biases. This has shifted the burden on companies to actively counteract bias.
A similar shift needs to happen with regard to AI’s accuracy. Companies deploying AI need to improve their standards for public reporting and allow external audits to evaluate stated accuracy claims. The burden of proof needs to shift to the company to affirmatively show that their AI systems work before they can be deployed.
Change starts with each of us. On this blog, we’ll give you ideas on how to resist AI snake oil in your own way — in the way you interact with AI products and vendors, read news articles, or evaluate your elected representatives and their approach to the AI industry.
I appreciate your article from my personal experience in an ongoing horror story; one that seems controlled by an Ai company's control over their corporate customers. This 3rd party company does pre-screening for potential tenants in hundreds of apartment complexes across the U.S. It is incredulous to me that these large landlords allow a non-fiduciary to make decisions that affect their bottom line, all without oversight.
My spotless record of rental history, no criminal history, and no credit history (I'm sorry, I don't borrow money nor do I owe anyone) has been targeted by the Ai to deny me rental in several rent applications.
There is no human to give me answers, only that "they "are required to go by what the Ai tells them without question." What if the Ai is crazy af? I''ve never once experienced the slightest hesitation in getting a rental before. This is as incredulous as it is futile.
Disputing the Ai's data and decision to deny me rent, calls to property managers, and an inability to reach human managers who purposely hide from consumers... has gotten me nowhere. It has now been six weeks with me living out of a suitcase, jumping from hotel to airbnb in a futile attempt to find a place to rent and call home. I'm going broke for no reason whatsoever? Attorneys wil not take my case, and I'm left frustrated and baffled, without answers. It's heinous! It's infuriating and stupid. It is ludicrous to let Ai ruin lives like this. Sad to be living in these awful times...
Thanks for starting this newsletter and looking forward to the book.
What is missing for me (at least for now) in this sneak peek is the connection with social and behavioural sciences. And some historical background. I know Dr Narayanan
and others are working with social science experts on this so that is definitely the right approach. I hope the book project will reflect that.
The narrative used by the media is one of the components. I did a short piece on this in my newsletter (https://sadnewsletter.substack.com/p/from-snake-oil-to-theranos) a while back to think about snake oil and myth from a socio-religious-historical perspective. Yes, the power of media helps in perpetuating the myth and we are dazzled by the hype. But there's some structural and historical basis for it. I agree that like the bias issue "a similar shift needs to happen about AI’s accuracy" and of course "Companies deploying AI need to improve their standards for public reporting". But we still considerable investor attention to such AI hypes. Media is amplifying no doubt but that is not happening in a vacuum.
Great work!