On the Societal Impact of Open Foundation Models
Adding precision to the debate on openness in AI
This post is authored by Sayash Kapoor, Rishi Bommasani, Daniel E. Ho, Percy Liang, and Arvind Narayanan. The paper has 25 authors listed here.
Last October, President Biden signed the Executive Order on Safe, Secure, and Trustworthy AI. It tasked the National Telecommunications and Information Administration (NTIA) with preparing a report on the benefits and risks of open foundation models—foundation models with widely available model weights (such as Meta's Llama 2 and Stability's Stable Diffusion). There is widespread disagreement about the impact of openness on society, which the NTIA must sort through. Last week, the NTIA released a list of over 50 questions to solicit public input on the benefits and risks of open foundation models. The responses the NTIA receives will inform its report, which will, in turn, influence U.S. policy on open foundation models.
Today, we are releasing a paper on the societal impact of open foundation models. We make three main contributions. First, we diagnose that the disagreement on the impact of openness results from a lack of precision in claims about its societal impact. Second, we analyze the benefits of open foundation models such as transparency, distribution of power, and enabling scientific research (including when open model weights are not enough to realize some benefits). Third, we offer a risk assessment framework for assessing the marginal risk of open foundation models compared to closed models or existing technology like web search on the internet.
The paper is the result of a collaboration with 25 authors across 16 academic, industry, and civil society organizations. Our aim is to bring clarity to pressing questions about how foundation models should be released and propose paths forward for researchers, developers, and policymakers.
Background: The debate on open foundation models
One of the biggest tech policy debates today is about how foundation models should be released. Access to some foundation models like OpenAI’s GPT-4 is limited to an API or a developer-provided product interface like ChatGPT. We call these models closed. Others, such as Meta’s Llama 2, are open, with widely available model weights enabling downstream modification and scrutiny. The United States, EU, and UK are all actively considering how to regulate open foundation models.
While there are many axes involved in releasing models that form a gradient, we focus on the dichotomy based on whether the weights are released widely. In particular, many of the risks described for open foundation models arise because developers relinquish control over who can use the model once it is released. For example, restrictions on what a model can be used for are both challenging to enforce and easy for malicious actors to ignore. In contrast, developers of closed foundation models can, in theory, reduce, restrict, or block access to their models. In short, the open release of model weights is irreversible.
As a result of this widespread access, some argue that widely available model weights could enable better research on their effects, promote competition and innovation, and improve scientific research, reproducibility, and transparency. Others argue that widely available model weights would enable malicious actors to more effectively misuse these models to generate disinformation, non-consensual intimate imagery, scams, and bioweapons.
Toward more precise analyses of societal impact
In October 2023, researchers from MIT released a study on the use of open language models for creating bioweapons. They looked at what information a malicious user might be able to find using open models that could aid the creation of pandemic-causing pathogens. Their findings were ominous, and their main recommendation to policymakers was to essentially ban open foundation models:
Policymakers should recognize that it is not possible to stop third parties from removing safeguards from models with publicly accessible weights. Avoiding model weight proliferation appears to be necessary – but not sufficient – to prevent a future in which highly capable artificial intelligence can be abused to cause mass harm.
While the paper focused on what information about building bioweapons users could find using language models, it did not compare it with information widely available on the internet. Follow-up studies from the RAND Institute and OpenAI focused on comparing the biosecurity risk from language models to information widely available on the internet. In stark contrast to the paper's claims, neither the RAND nor the OpenAI studies found that participants who used language models were significantly better than those who only used the internet to find information required to create bioweapons.
The MIT study is a cautionary tale of what can go wrong when analyses of the risks of open foundation models do not compare against risks from closed models or existing technology (such as web search on the internet). It also shows how in the absence of a clear methodology for analyzing the risks of open foundation models, researchers can end up talking past each other.
In our paper, we present a risk assessment framework to analyze the marginal risk of open foundation models. We focus on the entire pipeline of how societal risk materializes, rather than narrowly focusing on the role of AI and open foundation models. This expands the scope of possible interventions, such as protecting downstream attack surfaces. The framework is based on the threat modeling framework in cybersecurity and consists of six steps to analyze the risk of open foundation models:
Threat identification: Specify what the threat is and who it's from. For example, in cybersecurity, there are many potential risks from open foundation models, such as automated vulnerability detection and malware generation. Similarly, the marginal risk analysis would be very different if the risk is from individuals or small groups vs. heavily resourced groups such as state-sponsored attackers.
Existing risk (absent open foundation models). In many cases, the risk of releasing models openly already exists in the world (though perhaps at a different level of severity). What is the existing risk of this threat?
Existing defenses (absent open foundation models). Similarly, many purported risks of open foundation models have existing defenses. For example, there are many existing defenses to the risk of phishing email scams. Email providers scan emails and senders' network traffic to prevent phishing and operating systems detect and warn users about malware downloaded from the internet.
Marginal risk. Once the threat vector, existing risk level, and scope of existing defenses are clear, it is important to understand the marginal risk of releasing models openly—compared to existing technology such as the internet, as well as compared to releasing closed foundation models.
Ease of defense against marginal risk. While existing defenses provide a baseline for addressing new risks introduced by open foundation models, new defenses can be implemented, or existing defenses can be modified to address the increase in overall risk. How easy is it to build or bolster defenses in response to the marginal risk?
Uncertainty and assumptions. Finally, some disagreements might stem from unstated assumptions by different researchers about the open foundation model ecosystem. The framework asks researchers to specify the uncertainties and assumptions implicit in their analysis, to clarify disagreements between researchers with different viewpoints.
In the paper, we give examples of how the framework can be used by looking at cybersecurity risks stemming from automated vulnerability detection and the risk of non-consensual deepfakes. For the former, we find that the current marginal risk of open foundation models is low and there are many approaches to defending against the marginal risk, including using AI for defense. For the latter, open foundation models pose considerable marginal risk at present, and plausible defenses seem hard.
The framework also helps clarify disagreements in past studies by revealing the different assumptions about risk. In fact, when we analyze studies across seven sources of risks against our framework, we find many of them lacking, and we hope the framework helps foster more constructive debate going forward. In particular, we encourage more grounded research on characterizing the marginal risk, especially as both model capabilities and societal defenses evolve: evidence of minimal marginal risk today should not be seen as absolute evidence that risks may not arise in the future as underlying assumptions change.
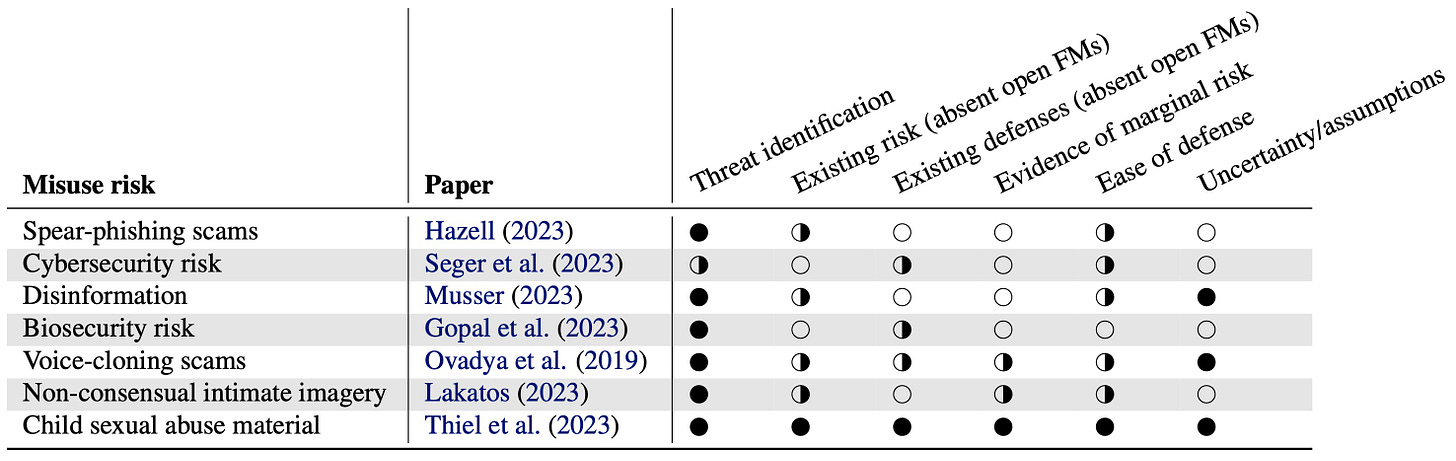
We also analyze the benefits of open foundation models. We first look at key properties of openness: broader access (by allowing a wide range of people to access model weights), greater customizability (by allowing users to tune models to their needs), local adaptation and inference (users can run open models on the hardware of their choice) and an inability to rescind access (foundation model developers cannot revoke access easily once released).
These properties lead to many benefits (with some caveats):
Distributing who defines acceptable model behavior: Broader access to models and their greater customizability expands who is able to specify the boundary of acceptable model behavior, instead of this decision-making power lying solely with foundation model developers.
Increasing innovation. Broader access, greater customizability, and local inference expand how foundation models are used to develop applications. For example, applications with strict privacy control requirements can use foundation models that run locally.
Enabling scientific research. Many types of research on and using foundation models require access to model weights. In the last two years, we have already seen examples of increasing speed and outlining safety challenges enabled by open models. At the same time, access to other assets such as data, documentation, and model checkpoints is necessary for other kinds of research, so providing model weights alone is not a panacea.
Enabling transparency. Broad access to weights enables some forms of transparency—for example, details about the model architecture. However, similar to research, transparency also requires assets other than model weights, notably public documentation, which is often lacking even when model weights are released openly.
Mitigating monoculture and market concentration. The use of the same foundation model across different applications leads to monoculture. When the model fails, or something goes wrong, it then affects all of these downstream applications. Greater customizability mitigates some of the harms of monoculture since downstream users of foundation models can fine tune them to change their behavior. Similarly, broader access to models could help reduce market concentration in the downstream market by lowering the barrier to entry for developing different types of foundation models. At the same time, open foundation models are unlikely to reduce market concentration in the highly concentrated upstream markets of computing and specialized hardware providers.
Recommendations for developers, researchers, regulators, and policymakers
Our analysis of benefits and risks opens up concrete next steps for a wide range of stakeholders. In particular:
Developers of open foundation models should clarify the division of responsibility between them and the downstream users of the product. In particular, developers should clarify which responsible AI practices are implemented and which ones are left for downstream users who might modify the model for use in a consumer-facing application.
Researchers investigating the risks of open foundation models should adopt the risk assessment framework to clearly articulate the marginal risk of releasing foundation models openly. Without such an assessment, it is unclear if the risks being outlined are also present in the status quo (i.e., without the release of open models) or if open models genuinely pose new risks that we cannot develop good defenses for.
Policymakers should proactively assess the impact of proposed regulation on open foundation models, especially in the absence of authoritative evidence of their marginal risk. Funding agencies should ensure that research investigating the risks of open foundation models is sufficiently funded while remaining appropriately independent from the interests of foundation model developers.
Competition regulators should invest in measuring the benefits of foundation models and the impact of openness on those benefits more systematically.
We aim to rectify the conceptual confusion around the impact of openness by clearly identifying their distinctive properties and clarifying their benefits and risks. While some philosophical tensions surrounding the release strategies for open foundation models will probably never be resolved, we hope that our conceptual framework helps address today's deficits in empirical evidence.
Further reading
The genesis of the paper was from a workshop we organized last September on responsible and open foundation models. Watch the video here and read a summary of the event here.
In December 2023, we wrote a policy brief on open foundation models based on lessons from our work, which is cited in NTIA's call for public input.
Our recent efforts are built on our prior work on release norms for foundation models, policy recommendations for AI regulation, limitations of licensing AI development, and analyses of the Executive Order’s impact on openness.
Fascinating read, thanks for sharing!
I'm trying not to oversimplify things here, but it feels like we're at a point where the genie is already out of the bottle, right? Sure, there's room for government action to rein things in or soften the impact – and the framework you've laid out is super valuable for that. What I'm really pondering is whether we've reached a stage where we should just assume that any new tech is bound to spread everywhere online sooner or later. Whether it's companies from other countries (like Mistral) or even state players getting involved, the incentive to push boundaries seems inevitable.
Thanks again!
An interesting initiative, Sayash.
I am intrigued by your choice to develop and adopt a new risk assessment ‘methodology’. I tried to review your (many) co-authors and I could have missed it - but setting aside the impressive academic pedigrees - did your group include anyone with risk analysis and mitigation competencies, background, or experience? Thank you in advance.