With the release of OpenAI’s latest model o3, there is renewed debate about whether Artificial General Intelligence has already been achieved. The standard skeptic’s response to this is that there is no consensus on the definition of AGI. That is true, but misses the point — if AGI is such a momentous milestone, shouldn’t it be obvious when it has been built?
In this essay, we argue that AGI is not a milestone. It does not represent a discontinuity in the properties or impacts of AI systems. If a company declares that it has built AGI, based on whatever definition, it is not an actionable event. It will have no implications for businesses, developers, policymakers, or safety. Specifically:
Even if general-purpose AI systems reach some agreed-upon capability threshold, we will need many complementary innovations that allow AI to diffuse across industries to realize its productive impact. Diffusion occurs at human (and societal) timescales, not at the speed of tech development.
Worries about AGI and catastrophic risk often conflate capabilities with power. Once we distinguish between the two, we can reject the idea of a critical point in AI development at which it becomes infeasible for humanity to remain in control.
The proliferation of AGI definitions is a symptom, not the disease. AGI is significant because of its presumed impacts but must be defined based on properties of the AI system itself. But the link between system properties and impacts is tenuous, and greatly depends on how we design the environment in which AI systems operate. Thus, whether or not a given AI system will go on to have transformative impacts is yet to be determined at the moment the system is released. So a determination that an AI system constitutes AGI can only meaningfully be made retrospectively.
Nuclear weapons as an anti-analogy for AGI
Achieving AGI is the explicit goal of companies like OpenAI and much of the AI research community. It is treated as a milestone in the same way as building and delivering a nuclear weapon was the key goal of the Manhattan Project.
This goal made sense as a milestone in the Manhattan Project for two reasons. The first is observability. In developing nuclear weapons, there can be no doubt about whether you’re reached the goal or not — an explosion epitomizes observability. The second is immediate impact. The use of nuclear weapons contributed to a quick end to World War 2. It also ushered in a new world order — a long-term transformation of geopolitics.
Many people have the intuition that AGI will have these properties. It will be so powerful and humanlike that it will be obvious when we’ve built it. And it will immediately bring massive benefits and risks — automation of a big swath of the economy, a great acceleration of innovation, including AI research itself, and potentially catastrophic consequences for humanity from uncontrollable superintelligence.
In this essay, we argue that AGI will be exactly the opposite — it is unobservable because there is no clear capability threshold that has particular significance; it will have no immediate impact on the world; and even a long-term transformation of the economy is uncertain.
In previous essays, we have argued against the likely disastrous policy interventions that some have recommended by analogizing AGI to nuclear weapons. It is striking to us that this analogy reliably generates what we consider to be incorrect predictions and counterproductive recommendations.
It isn’t crazy to think that o3 is AGI, but this says more about AGI than o3
Many prominent AI commentators have called o3 a kind of AGI: Tyler Cowen says that if you know AGI when you see it, then he has seen it. Ethan Mollick describes o3 as a jagged AGI. What is it about o3 that has led to such excitement?
The key innovation in o3 is the use of reinforcement learning to learn to search the web and use tools as part of its reasoning chain.1 In this way, it can perform more complex cognitive tasks than LLMs are directly capable of, and can do so in a way that’s similar to people.
Consider a person doing comparison shopping. They might look at a few products, use the reviews of those products to get a better sense of what features are even important, and use that knowledge to iteratively expand or shrink the set of products being considered. o3 is a generalist agent that does a decent job at this sort of thing.
Let’s consider what this means for AGI. To avoid getting bogged down in the details of o3, imagine a future system whose architecture is identical to o3, but is much more competent. For example, it can always find the right webpages and knowledge for the task as long as it’s online, no matter how hard it is to locate. It can download and run code from the internet to solve a task if necessary. None of these require scientific breakthroughs, only engineering improvements and further training.
At the same time, without scientific improvements, the architecture imposes serious limits. For example, this future system cannot acquire new skills from experience, except through an explicit update to its training. Building AI systems that can learn on the fly is an open research problem.2
Would our hypothetical system be AGI? Arguably, yes. What many AGI definitions have in common is the ability to outperform humans at a wide variety of tasks. Depending on how narrowly the set of tasks is defined and how broadly the relevant set of humans for each task is defined, it is quite plausible that this future o3-like model/agent will meet some of these AGI definitions.
For example, it will be superhuman at playing chess, despite the fact that large language models themselves are at best mediocre at chess. Remember that the model can use tools, search the internet, and download and run code. If the task is to play chess, it will download and run a chess engine.
Despite human-level or superhuman performance at many tasks, and plausibly satisfying some definitions of AGI, it will probably fail badly at many real-world tasks. We’ll get back to the reasons for that.
Does any of this matter? It does. Leaders at AI companies have made very loud predictions and commitments to delivering AGI within a few years. There are enormous incentives for them to declare some near-future system to be AGI, and potentially enormous costs to not doing so. Perhaps some of the valuation of AI companies is based on these promises, so without AGI there might be a bubble burst. Being seen as leaders in AI development could help improve market share and revenues, and improve access to talent.
So, if and when companies claim to have built AGI, what will be the consequences? We'll analyze that in the rest of this essay.
AGI won't be a shock to the economy because diffusion takes decades
One argument for treating AGI as a milestone — and taking declarations of AGI seriously — is that AGI could lead to rapid economic impacts, both positive and negative, such as a world without scarcity, an end to the concept of money, or sudden mass joblessness.
But AI's economic impact is only realized when it is adopted across the economy. Technical advances are necessary, but not sufficient, to realize this impact. For past general-purpose technologies, such as electricity, computing, and the internet, it took decades for the underlying technical advances to diffuse across society. The miracle of the Industrial Revolution wasn't the high growth rate — annual growth rates averaged below 3% — but the sustained period of decades of growth.
There are many bottlenecks to the diffusion of AI: developing useful products and applications, training the workforce to utilize these products, implementing organizational changes to enable AI use, and establishing laws and norms that facilitate AI adoption by companies. Like past general-purpose technologies, we expect the economic impacts of AI to be realized over decades, as this process of diffusion unfolds.
In the paper AI as Normal Technology, we present a detailed argument for why we think this will be the case. The idea that rapid increases in capability lead to rapid economic impacts is completely inconsistent with the past and present of AI, and there is no reason to expect that this will change in the future.
One definition of AGI is AI systems that outperform humans at most economically valuable work. We might worry that if AGI is realized in this sense of the term, it might lead to massive, sudden job displacement. But humans are a moving target. As the process of diffusion unfolds and the cost of production (and hence the value) of tasks that have been automated decreases, humans will adapt and move to tasks that have not yet been automated. The process of technical advancements, product development, and diffusion will continue.
AGI will not lead to a rapid change in the world order
The US and China are often described as being in an AI arms race, with each country racing to build AGI. It is hypothesized that the country to build it first would have a decisive strategic advantage — resulting in dominance in the world order for the foreseeable future.3
This narrative doesn’t make sense because the knowledge required to create AI models, and model capabilities themselves, tend to proliferate quickly between countries. There are hundreds of thousands of AI technologists, and they work in the private sector rather than government labs, so it is not feasible to keep secrets at that scale.
Invention — in this case, AI model development — is overrated as a source of competitive advantage. We should expect technological developments to roughly keep pace across countries. Even though US companies are currently at the forefront, we shouldn't expect a lasting advantage.4
Many people haven't appreciated the ease of proliferation of technological capabilities — perhaps due to the nuclear weapons mental model — and have been caught by surprise. This is what led to the "DeepSeek moment" earlier this year, since analysts did not realize how quickly AI capabilities can proliferate, and as a result, were not expecting upstarts (especially from China) to catch up so quickly.
Some people argue that even an advantage of a few months will be critical. We disagree. The important question in the context of great power competition is not which country builds AGI first, but rather which country better enables diffusion. As Jeffrey Ding has shown, the effectiveness of companies and governments in actually utilizing AI inventions and innovations, whether domestic or international, to improve productivity is far more important for determining the economic impacts of general-purpose technologies.
While Chinese AI companies are at most 6-12 months behind leading US companies in terms of AI models and capabilities, China lags significantly behind the US in several key indicators that might enable diffusion: Digitization, cloud computing adoption, and workforce training. All of these are required to enable the productive diffusion of AI advances across industries. This is the actual source of American competitive advantage.
Of course, this could change in the coming years. But if it does, it will result from policy changes to promote diffusion rather than the development of AGI. And it is not something countries can accomplish overnight, regardless of how quickly they change policy. Diffusion typically unfolds over decades.
None of this means that policymakers should be complacent. But it does mean that rather than fixating on AGI, they should focus on enabling productive and safe diffusion, including of existing AI.
The long-term economic implications of AGI are uncertain
Even if it doesn’t have immediate economic impacts, could AGI unlock, say, 10% annual GDP growth that could add up to something big over a few decades?
Maybe. But it is far from clear why and how this will happen.
Historically, this kind of acceleration in growth has happened very few times — the industrial revolution had this effect, but not the internet, which barely had any impact on GDP. Note that even if you don’t think that GDP is the right thing to measure, a qualitative change in the GDP growth rate is a good proxy for whatever fundamental change in the economy you care about.
The problem is that accelerating growth requires eliminating bottlenecks to progress. That’s harder than most AI boosters assume. AI will likely have uneven effects across sectors, and long-term growth will be bottlenecked by the weakest sector.
Those arguing for dramatic effects often have an incorrect mental model of what the bottlenecks actually are. For example, while it is tempting to believe that cheap scientific innovation will unlock progress, the production of new findings is actually not the bottleneck in science.
More broadly, progress depends not just on the technology but on having the right preconditions — complementary innovations as well as cultural, economic, and political factors. If all it took to create the industrial revolution was the invention of steam power, the Roman Empire would have done it.
Our current laws, norms, institutions, and politics evolved in a time of much less technological potential. They are already choking opportunities for straightforward types of growth, such as building more public infrastructure. To reap the economic benefits that broad cognitive automation can potentially bring, the degree of structural change that needs to happen is unfathomably greater.
In conclusion, the extent and nature of long-term impacts from AGI remain to be seen and depend on what complementary actions we take. The long-term implications are not a property of AGI itself.
Misalignment risks of AGI conflate power and capability
On the flip side, AGI could be a turning point for AI's societal risks. Could it cause loss of control, massive societal harm, or even human extinction?
Discussions of AGI risks conflate power — the ability to modify the environment — with capability — the capacity to solve specified tasks correctly. Capability is an intrinsic property of an AI system, whereas power is a matter of how we design the environment in which AI systems operate. And humans have agency over this design. This distinction is often overlooked.
Consider Dario Amodei's definition of "powerful AI".5 He begins with a description of the capabilities of powerful AI, such as being able to "...prove unsolved mathematical theorems, write extremely good novels, write difficult codebases from scratch." This criterion is an example of AI capabilities, and we can discuss them at the level of AI systems.
But he then moves on to describe properties of the environment in which we allow AI systems to operate, which includes "...taking actions on the internet, taking or giving directions to humans, ordering materials, directing experiments, watching videos, making videos, and so on." This is an example of the power afforded to an AI system. It depends on the environment within which the AI system operates, which determines how AI capabilities are translated into power.
We do expect AI capabilities to keep increasing. But regardless of capability level, we can choose to ensure that AI remains a tool and is not given power and autonomy to operate without human oversight. In the AI as Normal Technology essay, we address all the usual counterarguments to this, including arms races among companies, power seeking, superhuman persuasion, deceptive alignment, and more.
We argue in the paper that there will be strong business incentives against deploying AI without adequate oversight, and that these incentives can and should be buttressed by regulation when necessary. This has historically been the case in areas ranging from self-driving cars to AI assistants. We don’t expect this trend to suddenly flip once AI capabilities reach a presumed tipping point that we arbitrarily designate as AGI.
AGI does not imply impending superintelligence
Yet another reason to consider AGI a milestone is the view that shortly after we build AGI, AI systems could recursively self-improve — AGI could train future versions of models that become far more capable, leading to an "intelligence explosion." Soon afterwards, we would get superintelligent AI (AI systems that far exceed human abilities on any conceivable task), leading to either utopia or dystopia, depending on how well superintelligent AI is “aligned” with human interests.
In the normal technology view, there are two big reasons to doubt this narrative. The first is that even if arbitrary speedups in AI methods are possible, we think that innovation and diffusion will happen at human speed, as summarized in this table.
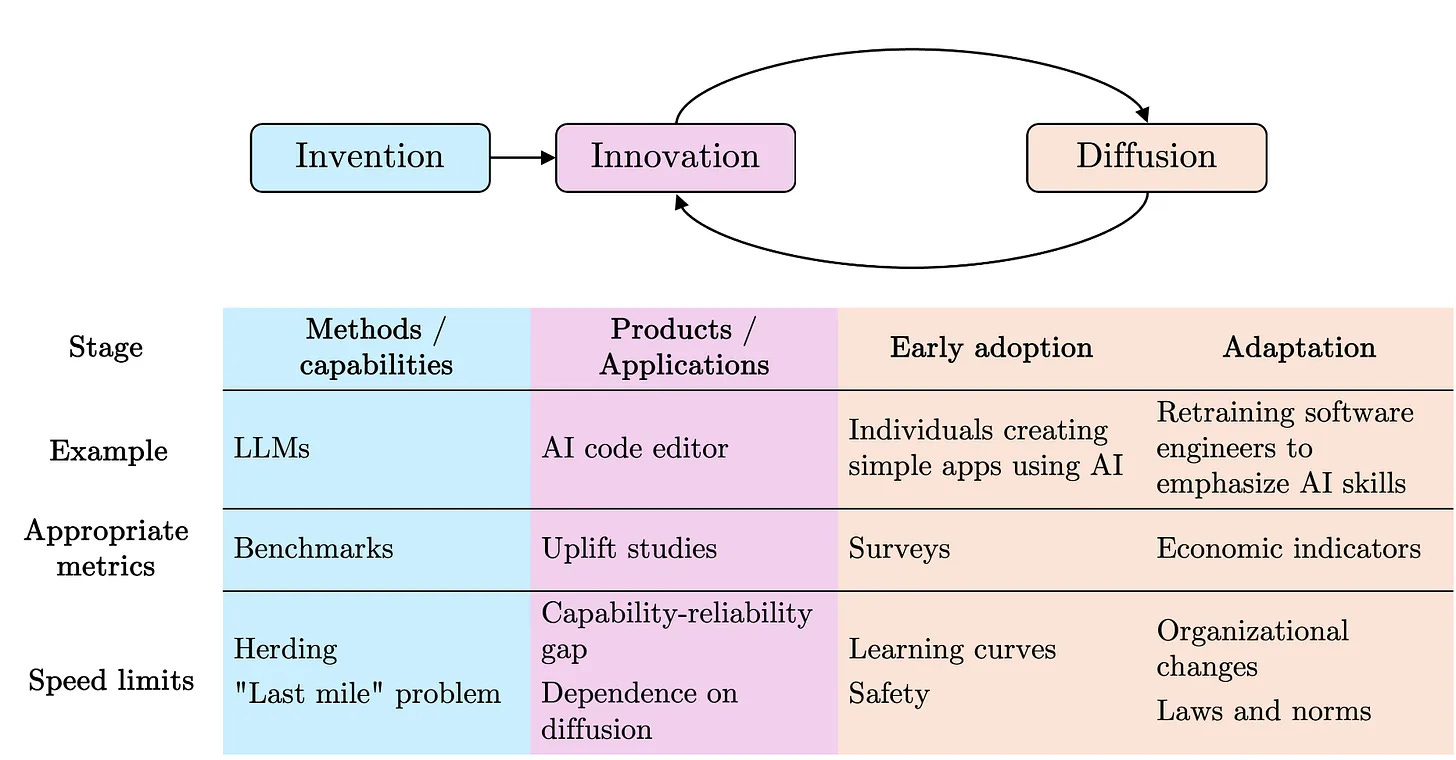
Second, the fact that AI would help conduct AI research does not imply that this process can be arbitrarily accelerated. AI is already used to automate a significant portion of AI research today. But there are many bottlenecks to progress in AI methods, such as the social nature of data collection and real-world interaction that might be required for achieving certain capabilities, computational and cost limits, or herding around popular or intuitive ideas while ignoring the ones that enable true breakthroughs.
We could be wrong about this, and recursive self-improvement could be possible, leading to unbounded speedups in progress in AI methods. And this might have some interesting implications, including some discontinuities in impact, even if widespread diffusion will be slower. For these reasons, it is important to have early warning systems for recursive self-improvement. However, this is not captured by AGI definitions. We might well have AGI while being far from recursive self-improvement, or vice versa.
We won’t know when AGI has been built
There are endless definitions of AGI and related concepts — Jasmine Sun has a useful compilation of over 20 definitions — but they fall into three broad categories: they can be based on the system’s impact on the world, its internals, or its behavior in controlled settings. We will show that each style of definition has a fatal flaw. It leads to criteria that are either too strict or too weak compared to what we ideally want.
Understanding these gaps also shows why people have different intuitions regarding what AGI will look like, and why “we’ll know it when we see it” has failed as a criterion and will continue to fail.
OpenAI's 2018 definition of AGI was "highly autonomous systems that outperform humans at most economically valuable work". From our perspective — our interest being in the impacts of AI — this definition is potentially very useful. If AI outperformed [all] humans at most economically valuable work, it would be unquestionably impactful.
But let’s be clear — this is not a property of an AI system. It is a property of the state of the world. It has at least as much to do with the complementary innovations that we make and the extent to which we choose to integrate AI into our organizations and institutions. It would be absurd to try to test an AI system in isolation in the lab and ask whether it outperforms people at their jobs. It is a category error.
For example, whether AI can (autonomously) outperform a medical researcher depends in part on whether we collectively choose to allow AI systems to perform large-scale medical experiments on people. We shouldn’t and we won’t, which means that irrespective of the systems' capabilities, they cannot perform the function of a medical researcher. This might be an extreme example, but similar bottlenecks arise in virtually every job.
Worse, until and unless we diffuse AI everywhere, we won’t even know if the system is theoretically capable of automating work in the real world. We won’t be able to set up sufficiently convincing simulacra of the messy complexity of the world. In short, impact-based definitions are not useful for practical purposes because they don’t give us a way to anticipate the end result of the painfully slow process of diffusion.
In contrast to researchers like us who are interested in impacts, many researchers are interested in humanlike AI in the sense of internals — does the system truly understand the world causally, can it reason, plan, and acquire new skills like we do, and so forth.
This sense of AGI has been notoriously hard to operationalize because of the difficulty of observing and characterizing AI internals. The Turing test is the best known of many attempts to use behavior as a proxy for the humanlike attributes we care about, but inevitably it turns out that we can build AI systems that pass such tests without having the hoped-for humanlike internals.
Furthermore, because of the jaggedness of AI — superhuman in many ways, yet lacking a toddler’s understanding of the world in other ways — the transformative effects of AI are likely to be felt long before it is fully humanlike (or superhuman) on all dimensions.
In short, since we are not interested in the internals for their own sake, we set aside this kind of definition.
That leaves us with the third kind of definition, which is by far the most common: those based on behavior, operationalized as benchmark performance. For example, the Metaculus question on “human-machine intelligence parity” is defined in terms of performance on exam questions in math, physics, and computer science. The problem with this kind of definition is well known, and we’ve discussed it repeatedly. They simply encourage hill climbing in the sense of building AI systems that can beat the benchmarks without necessarily being useful in the real world.
One response to the definitional challenge is to claim that we'll know AGI when we see it. The o3 launch shows that the opposite is true — to some people, it is clear that the advances in capabilities represent a step change that warrants calling it AGI. To others, the improvements are marginal at best, and unlikely to lead to real-world impact.
What explains people’s differing intuitions? Here’s our guess. While AI capabilities can be general, making AI useful in the real world will have to happen in a largely domain-specific way. (The generalist agent aspect of o3 is misleading — it can only handle generative tasks where errors have low cost, not when you need it to operate independently in the real world. For example, a useful travel booking AI agent hasn’t yet been released, despite the seeming triviality of the task for humans.)
So when people are thinking about whether o3 or any other system is (close to) AGI, they are intuitively thinking about different domains, and the gap between general capabilities and useful real-world abilities is vastly different from one domain to another. Crossing the threshold of having useful products that can automate most tasks may happen at vastly different times in different sectors or jobs.
Businesses and policy makers should take a long-term view
AGI is not a milestone because it is not actionable. A company declaring it has achieved, or is about to achieve, AGI has no implications for how businesses should plan, what safety interventions we need, or how policymakers should react. What should businesses and policy makers do instead?
Businesses should not rush to adopt half-baked AI products. Rapid progress in AI methods and capabilities does not automatically translate to better products. Building products on top of inherently stochastic models is challenging, and businesses should adopt AI products cautiously, conducting careful experiments to determine the impact of using AI to automate key business processes. In particular, we are extremely skeptical of the idea of AI agents being “drop-in replacements” for human workers that will somehow bypass the need for careful evaluation and integration of automation into workflows.
Companies developing AI products need a deep understanding of the domain to identify hurdles to adoption and build what businesses adopting AI want. For example, one key innovation of AI-enabled code editors such as Cursor and Windsurf is the user interface which allows programmers to verify AI-generated text at different levels of abstraction. Other industries will have different obstacles to AI adoption that products should address.
Policy makers should also take a long term view. A “Manhattan Project for AGI” is misguided on many levels. Since AGI is not a milestone, there is no way to know when the goal has been reached or how much more needs to be invested. And accelerating AI capabilities does nothing to address the real bottlenecks to realizing its economic benefits.
This view also has implications for export controls. The US has applied export controls on the hardware needed for AI development, hoping to slow down Chinese AI development. Proponents of export controls admit (and we agree) that this will not widen the gap between the US and China by more than a few months. But this only matters in a world where the impacts of developing advanced AI are rapid. If the impact of advanced AI is realized through diffusion, and the process of diffusion takes decades, being ahead by a matter of months in a game of decades is of little consequence. Policy makers should therefore focus on enabling diffusion. We outline some ideas on how to do so in our recent essay.
Treating AGI as a milestone for the development of transformative AI is seductive but misguided. It feeds into incorrect mental models of AI progress and risks, economic impacts, and geopolitics. AI's impact on the world will be realized not through a sprint toward a magic-bullet technology but through millions of boring little business process adaptations and policy tweaks.
We are grateful to Steve Newman and Jasmine Sun for feedback on a draft of this essay.
Further reading
Jasmine Sun’s recent essay is similar to ours in spirit and substance. She contrasts many popular definitions of AGI and concludes that AGI is better seen as an aspiration for the AI community rather than a concrete technical milestone. She has also compiled popular definitions of AGI and other related concepts.
Borhane Blili-Hamelin and others argue that we should stop treating AGI as the North star for AI research.
Ege Erdil makes the case for multi-decade timelines to transformative AI capabilities.
Eryk Salvaggio gives many reasons why it is dangerous for policy makers to act as if AGI is imminent.
In AI as Normal Technology, we laid the intellectual foundation for our view of the future of AI. The present essay is the first of many follow-ups that explore the implications of the ideas therein.
While LLMs can only output text, models like o3 are trained to use their text outputs to interact with tools. For example, o3 can output a specific keyword (say, "use-search") that allows it to search for a given query online. To be clear, previous LLMs could also interact with tools. For example, OpenAI's ChatGPT plugins allowed models like GPT-4 to use tools two years ago. The key difference with models like o3 is that they are trained to use tools effectively. Previous models used tools based on prompts without explicitly being trained for this task, or at best were taught the syntax of tool use. o3 has access to many tools on the ChatGPT interface, including search, the ability to run code, access files, and generate and reason about images. Overall, o3 is much more like an "agent" than a chatbot.
For example, suppose this system is used to carry out a new type of coding task, perhaps using a software library that was only released after it finished training. Given enough documentation and instructions, it might eventually correctly perform the task. But barring an explicit update to the model via retraining, this "experience" of correctly performing the task would not change the model's weights, so another user needing to perform the same task would need to give it the same help that the first user did.
We do not analyze military AI in this piece. It is an important topic that we will return to in future essays.
It is important to distinguish between the invention of new technology and innovations that help those technologies diffuse across society; our discussion in this paragraph is about the former. Invention refers to the development of new models, perhaps accompanied by new capabilities. Innovations enable these capabilities to be used productively. Better and more capable models are an example of invention, whereas the development of products that use these models is an example of innovation. We don't think it is likely that countries will be able to maintain a long and sustained advantage in invention. But as we discuss later in this section, the conditions that enable the productive use of inventions can differ greatly between countries.
Amodei eschews AGI and uses the term “powerful AI” to be more precise about his predictions.
This article helped me breathe a bit easier, especially taking a step back and thinking how long diffusion will likely take. I have this (dramatic) schema in my mind that it'll happen overnight and we will all be found with our pants down (metaphorically).
Amazingly lucid and pouring cold water on hype and fear.
AGI will be just one ingredient in the mix. The rate of change is, as always, dependent on the slowest component.