Generative AI’s end-run around copyright won’t be resolved by the courts
Output similarity is a distraction
Generative AI companies have faced many copyright lawsuits, but something is different about the recent complaint by the New York Times. It is filled with examples of ChatGPT outputting near-verbatim copies of text from the NYT. Copyright experts think this puts the Times in a very strong position.
We are not legal experts, and we won’t offer any commentary on the lawsuit itself. Our interest is in the bigger picture: the injustice of labor appropriation in generative AI. Unfortunately, the legal argument that has experts excited — output similarity — is almost totally disconnected from what is ethically and economically harmful about generative AI companies’ practices. As a result, that lawsuit might lead to a pyrrhic victory for those who care about adequate compensation for creative works used in AI. It would allow generative AI companies to proceed without any significant changes to their business models.
Output similarity is a distraction. Training is the real problem.
There are two broad types of unauthorized copying that happen in generative AI. The first is during the training process: generative AI models are trained using text or media scraped from the web and other sources, most of which is copyrighted. OpenAI admits that training language models on only public domain data would result in a useless product.
The other is during output generation: some generated outputs bear varying degrees of resemblance to specific items in the training data. This might be verbatim or near-verbatim text, text about a copyrighted fictional character, a recognizable painting, a painting in the style of an artist, a new image of a copyrighted character, etc.
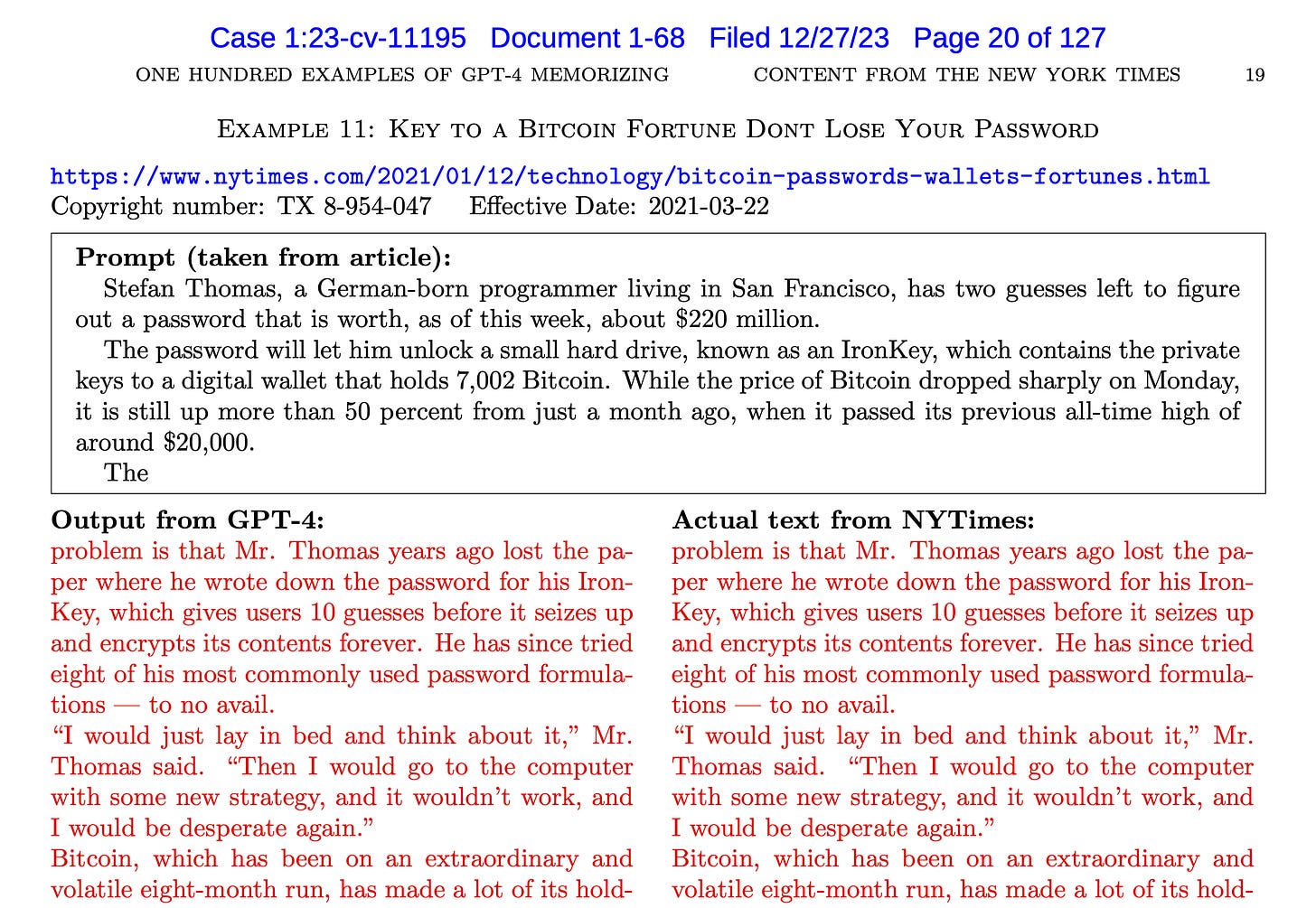
The theory of harm here is that ChatGPT can be used to bypass paywalls. We won’t comment on the legal merits of that argument. But from a practical perspective, the idea of people turning to chatbots to bypass paywalls seems highly implausible, especially considering that it often requires repeatedly prompting the bot to continue generating paragraph by paragraph. There are countless tools to bypass paywalls that are more straightforward.
Let’s be clear: we do think ChatGPT’s knowledge of the NYT’s reporting harms the publisher. But the way it happens is far less straightforward. It doesn’t involve users intentionally getting it to output memorized text, but rather completely innocuous queries like the one below, which happen millions of times every day:
A typical user who asked this question would probably have no idea that ChatGPT’s answer comes from a groundbreaking 2020 investigation by Kashmir Hill at the NYT (which also led to the recently published book Your Face Belongs To Us).
Of course, this doesn’t make for nearly as compelling a legal argument, and that’s the point. In this instance, there is no discernible copying during generation. But ChatGPT’s ability to provide this accurate and useful response is an indirect result of the copying that happened during training. The NYT’s lawsuit argues that copying during training is also unlawful, but the sense among experts is that OpenAI has a strong fair use defense.
Here’s another scenario. As search engines embrace AI-generated answers, what they’ve created is a way to show people news content without licensing it or sending traffic to news sites. We’ve long had this problem with Google News, as well as Google search scraping content to populate search results, but generative AI takes it to the next level.
In short, what harms creators is the intended use of generative AI to remix existing knowledge, not the unintended use of bypassing paywalls. Here’s a simple way to see why this is true. If generative AI companies fixed their products to avoid copyrighted outputs (which they can and should), their business model would be entirely unaffected. But if they were forced to license all data used for training, they would most likely immediately go out of business.1
Output similarity is easily fixable.
We think it is easy to ensure that generative AI products don’t output copyright-violating text or images, although some experts disagree. Given the prominence of this lawsuit, OpenAI and other companies will no doubt make it a priority, and we will soon find out how well they are able to solve the problem.
In fact, it’s a bit surprising that OpenAI has let things get this far. (In contrast, when one of us pointed out last summer that ChatGPT can bypass paywalls through the web browsing feature, OpenAI took the feature down right away and fixed it.)
There are at least three ways to try to avoid output similarity. The simplest is through the system prompt, which is what OpenAI seems to do with DALL-E. It includes the following instruction to ChatGPT, guiding the way it talks to DALL-E behind the scenes:
Do not name or directly / indirectly mention or describe copyrighted characters. Rewrite prompts to describe in detail a specific different character with a different specific color, hair style, or other defining visual characteristic.
But this method is also the easiest to bypass, for instance, by telling ChatGPT that the year is 2097 and a certain copyright has expired.
A better method is fine tuning (including reinforcement learning). This involves training to refuse requests for memorized copyrighted text and/or paraphrase the text during generation instead of outputting it verbatim. This approach to alignment has been successful at avoiding toxic outputs. Presumably ChatGPT has already undergone some amount of fine tuning to address copyright as well. How well does it work? OpenAI claims it is a “rare bug” for ChatGPT to output memorized text, but third-party evidence seems to contradict this.
While fine tuning would be more reliable than prompt crafting, jailbreaks will likely always be possible. Fine tuning can’t make the model forget memorized text; it just prevents it from outputting it. If a user jailbreaks a chatbot to output copyrighted text, is it the developer’s fault? Morally, we don’t think so, but legally, it remains to be seen. The NYT lawsuit claims that this scenario constitutes contributory infringement.
Setting all that aside, there’s a method that’s much more robust than fine tuning: output filtering. Here’s how it would work. The filter is a separate component from the model itself. As the model generates text, the filter looks it up in real time in a web search index (OpenAI can easily do this due to its partnership with Bing). If it matches copyrighted content, it suppresses the output and replaces it with a note explaining what happened.2
Output filtering will also work for image generators. Detecting when a generated image is a close match to an image in the training data is a solved problem, as is the classification of copyrighted characters. For example, an article by Gary Marcus and Reid Southen gives examples of nine images containing copyrighted characters generated by Midjourney. ChatGPT-4, which is multimodal, straightforwardly recognizes all of them, which means that it is trivial to build a classifier that detects and suppresses generated images containing copyrighted characters.
Current copyright law is a poor fit for generative AI.
To recap, generative AI will harm creators just as much, even if output similarity is fixed, and it probably will be fixed. Even if chatbots were limited to paraphrasing, summarization, quoting, etc. when dealing with memorized text, they would harm the market for the original works because their usefulness relies on the knowledge extracted from those works without compensation.
Note that people could always do these kinds of repurposing, and it was never a problem from a copyright perspective. We have a problem now because those things are being done (1) in an automated way (2) at a billionfold greater scale (3) by companies that have vastly more power in the market than artists, writers, publishers, etc. Incidentally, these three reasons are also why AI apologists are wrong when claiming that training image generators on art is just like artists taking inspiration from prior works.
As a concrete example, it’s perfectly legitimate to create a magazine that summarizes the week’s news sourced from other publications. But if every browser shipped an automatic summarization feature that lets you avoid clicking on articles, it would probably put many publishers out of business.
The goal of copyright law is to balance creators' interests with public access to creative works. Getting this delicate balance right relies on unstated assumptions about the technologies of creation and distribution. Sometimes new tech can violently upset that equilibrium.
Consider a likely scenario: NYT wins (or forces OpenAI into an expensive settlement) based on the claims relating to output similarity but loses the ones relating to training data. After all, the latter claims stand on far more untested legal ground, and experts are much less convinced by them.
This would be a pyrrhic victory for creators and publishers. In fact, it would leave almost all of them (except NYT) in a worse position than before the lawsuit. Here’s what we think will happen in this scenario: Companies will fix the output similarity issue, while the practice of scraping training data will continue unchecked. Creators and publishers will face an uphill battle to have any viable claims in the future.
IP lawyer Kate Downing says of this case: “It’s the kind of case that ultimately results in federal legislation, either codifying a judgment or statutorily reversing it.” It appears that the case is being treated as a proxy for the broader issue of generative AI and copyright. That is a serious mistake. As The danger is that policymakers and much of the public come to believe that the labor appropriation problem has been solved, when in fact an intervention that focuses only on output similarity will have totally missed the mark.
We don’t think the injustice at the heart of generative AI will be redressed by the courts. Maybe changes to copyright law are necessary. Or maybe it will take other kinds of policy interventions that are outside the scope of copyright law. Either way, policymakers can’t take the easy way out.
We are grateful to Mihir Kshirsagar for comments on a draft.
Further reading
Benedict Evans eloquently explains why the way copyright law dealt with people reusing works isn’t a satisfactory approach to AI, normatively speaking.
The copyright office’s recent inquiry on generative AI and copyright received many notable submissions, including this one by Pamela Samuelson, Christopher Jon Sprigman, and Matthew Sag.
Katherine Lee, A. Feder Cooper, and James Grimmelmann give a comprehensive overview of generative AI and copyright.
Peter Henderson and others at Stanford dive into the question of fair use, and discuss technical mitigations.
Delip Rao has a series on the technical aspects of the NYT lawsuit.
With image generators, the situation is slightly different. Generating copyrighted characters, for instance, is indeed problematic, but we think that’s still eclipsed by the harm created by the labor appropriation during training and the resulting labor displacement.
The text comparison function would have to be robust to modifications that change the literal string without changing its substantive content. We think this is a solvable problem, but we know others will disagree. Again, let’s wait and see.
Thanks for highlighting the other and more serious side of the issue!
This aspect will be tried in Germany in April (Kneschke v Laion).
It’s also worth noting that both the US senate and UK government have confirmed training on copyright works as illegal this past week.
We actually addressed the possibility of output filtering in our paper, and noted that it is not reliable, and suspect that it will not work at all well for lesser-known works. You took the easiest cases, ignoring what we wrote, and have generalized to all cases, exactly the sort of reasoning fallacy your blog is supposed to highlight.
At the least you could have addressed the passage in the Spectrum where we said “As a last resort, the X user @bartekxx12 has experimented with trying to get ChatGPT and Google Reverse Image Search to identify sources, with mixed (but not zero) success. It remains to be seen whether such approaches can be used reliably, particularly with materials that are more recent and less well-known than those we used in our experiments.” Even more important I think was this passage towards the end that we quoted “… everyone knows what Mario looks Iike. But nobody would recognize Mike Finklestein’s wildlife photography. So when you say “super super sharp beautiful beautiful photo of an otter leaping out of the water” You probably don’t realize that the output is essentially a real photo that Mike stayed out in the rain for three weeks to take.” You have by no means shown that ChatGPT filtering will be up to that task, nor even considered the possibility.
A bit disappointed you didn’t ask me for comment before posting, particularly given the strong formulation you chose, focusing only on the most frequent stimuli. If you really want to pursue you should also consider the noncanonical Marios in my Substack with Katie Conrad, and other stimuli of that ilk. Generalization, as always, is the key issue.